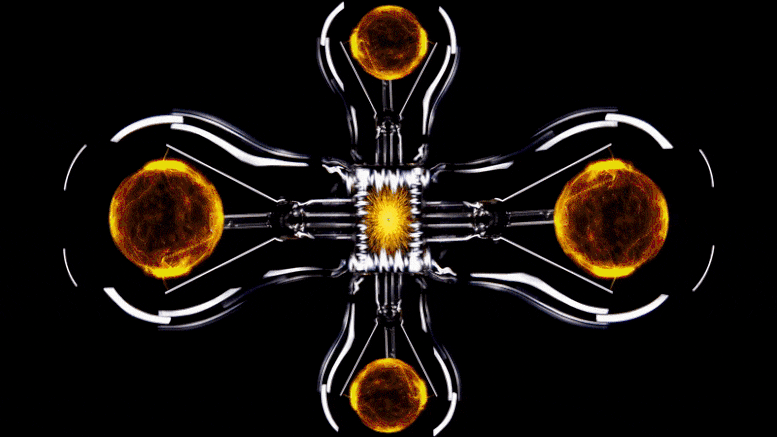
양자 물리학의 추상 그림입니다.
과학자들은 일반적으로 요구되는 것보다 훨씬 적은 수의 방정식을 사용하여 격자에서 움직이는 전자의 물리학을 포착하도록 기계 학습 도구를 훈련했습니다.[{” attribute=””>accuracy.
A daunting quantum problem that until now required 100,000 equations has been compressed into a bite-size task of as few as four equations by physicists using artificial intelligence. All of this was accomplished without sacrificing accuracy. The work could revolutionize how scientists investigate systems containing many interacting electrons. Furthermore, if scalable to other problems, the approach could potentially aid in the design of materials with extremely valuable properties such as superconductivity or utility for clean energy generation.
The study, by researchers at the Flatiron Institute and their colleagues, was published in the September 23 issue of Physical Review Letters.
“We start with this huge object of all these coupled-together differential equations; then we’re using machine learning to turn it into something so small you can count it on your fingers,” says study lead author Domenico Di Sante. He is an assistant professor at the University of Bologna in Italy and a visiting research fellow at the Flatiron Institute’s Center for Computational Quantum Physics (CCQ) in New York City.
The challenging quantum problem concerns how electrons behave as they move on a gridlike lattice. When two electrons occupy the same lattice site, they interact. Known as the Hubbard model, this setup is an idealization of several important classes of materials and enables scientists to learn how electron behavior gives rise to very sought-after phases of matter, including superconductivity, in which electrons flow through a material without resistance. The model also serves as a proving ground for new methods before they’re unleashed on more complex quantum systems.
A visualization of a mathematical apparatus used to capture the physics and behavior of electrons moving on a lattice. Each pixel represents a single interaction between two electrons. Until now, accurately capturing the system required around 100,000 equations — one for each pixel. Using machine learning, scientists reduced the problem to just four equations. That means a similar visualization for the compressed version would need just four pixels. Credit: Domenico Di Sante/Flatiron Institute
However, the Hubbard model is deceptively simple. For even a modest number of electrons and cutting-edge computational approaches, the problem requires massive computing power. That’s because when electrons interact, their fates can become quantum mechanically entangled. This means that even once they’re far apart on different lattice sites, the two electrons can’t be treated individually. Therefore, physicists are required to deal with all the electrons at once rather than one at a time. With more electrons, more entanglements crop up, making the formidable computational challenge exponentially harder.
“It’s essentially a machine that has the power to discover hidden patterns. When we saw the result, we said, ‘Wow, this is more than what we expected.’ We were really able to capture the relevant physics..” — Domenico Di Sante
One way of studying a quantum system is by using what’s called a renormalization group. That’s a mathematical apparatus physicists use to look at how the behavior of a system — such as the Hubbard model — changes when researchers modify properties such as temperature or look at the properties on different scales. Unfortunately, a renormalization group that keeps track of all possible couplings between electrons and doesn’t sacrifice anything can contain tens of thousands, hundreds of thousands, or even millions of individual equations that need to be solved. On top of that, the equations are quite tricky: Each represents a pair of electrons interacting.
Di Sante and his colleagues wondered if they could use a machine learning tool known as a neural network to make the renormalization group more manageable. The neural network is like a cross between a frantic switchboard operator and survival-of-the-fittest evolution. First, the machine learning program creates connections within the full-size renormalization group. The neural network then tweaks the strengths of those connections until it finds a small set of equations that generates the same solution as the original, jumbo-size renormalization group. The program’s output captured the Hubbard model’s physics even with just four equations.
“It’s essentially a machine that has the power to discover hidden patterns,” Di Sante says. “When we saw the result, we said, ‘Wow, this is more than what we expected.’ We were really able to capture the relevant physics.”
Training the machine learning program required considerable computational muscle, and the program ran for entire weeks. The good news, Di Sante says, is that now that they have their program coached, they can adapt it to work on other problems without having to start from scratch. He and his collaborators are also investigating just what the machine learning is actually “learning” about the system. This could provide additional insights that might otherwise be hard for physicists to decipher.
Ultimately, the biggest open question is how well the new approach works on more complex quantum systems such as materials in which electrons interact at long distances. In addition, there are exciting possibilities for using the technique in other fields that deal with renormalization groups, Di Sante says, such as cosmology and neuroscience.
Reference: “Deep Learning the Functional Renormalization Group” by Domenico Di Sante, Matija Medvidović, Alessandro Toschi, Giorgio Sangiovanni, Cesare Franchini, Anirvan M. Sengupta and Andrew J. Millis, 21 September 2022, Physical Review Letters.
DOI: 10.1103/PhysRevLett.129.136402
Di Sante co-authored the new study with CCQ guest researcher Matija Medvidović (a graduate student at Columbia University), Alessandro Toschi of TU Wien in Vienna, Giorgio Sangiovanni of the University of Würzburg in Germany, Cesare Franchini of the University of Bologna in Italy, CCQ and Center for Computational Mathematics senior research scientist Anirvan M. Sengupta, and CCQ co-director Andy Millis. Di Sante’s time at the CCQ was supported by a Marie Curie International Fellowship, which encourages transnational scientific collaboration.
“경순은 통찰력 있고 사악한 사상가로, 다양한 음악 장르에 깊은 지식을 가지고 있습니다. 힙스터 문화와 자연스럽게 어우러지는 그의 스타일은 독특합니다. 그는 베이컨을 좋아하며, 인터넷 세계에서도 활발한 활동을 보여줍니다. 그의 내성적인 성격은 그의 글에서도 잘 드러납니다.”